Intelligent Data Extraction: What, Why, and How
Today's post is from our friends at middleware.io, an AI-powered cloud observability platforms
"Data is the new oil." says the renowned Mathematician and Marketeer Clive Humby.
Every minute, businesses generate mammoth amounts of data from invoices, emails, and PDFs, to web pages. Research shows that enterprises spend over $30 billion manually extracting documents’ data. And manually sifting through this amount of data is like finding a needle in a haystack.
This is where intelligent data extraction steps in. It swiftly skims through the data and translates it instantly into digestible insights. Gartner reports that intelligent data extraction can save 25,000 hours of rework for the finance team caused by human error costing $878,000 annually for an enterprise with 40 members of an accounting team.
This blog post will discuss the what, why, and how behind this groundbreaking approach.
What Is Intelligent Data Extraction?
“Things get done only if the data we gather can inform and inspire those in a position to make a difference.” - Dr. Mike Schmoker, Author
At its core, Intelligent Data Extraction is born from the union of artificial intelligence and machine learning. With subsets of AI—like machine learning algorithms, natural language processing and many others—it dives deep into various data sources, be it printed documents, scanned images, or diverse electronic file formats.
It doesn’t just stop at extraction; it then routes the gleaned data through suitable channels. This ensures that the right nuggets of information meet the right eyes or workflows.
Picture this: In a healthcare setup, patient feedback is paramount. Intelligent data extraction combs through heaps of feedback forms isolates critical remarks, and feeds them directly to the relevant departments for swift action.
Industries across the board—from finance to retail—are tapping into its power. Whether it's discerning patterns in contracts or gleaning insights from customer feedback, intelligent data extraction promises accuracy, speed, and reduced error—ushering in a data-driven future.
Why Is Intelligent Data Extraction Important?
"It is a capital mistake to theorize before one has data."
Before data analysis, before predictions, and before strategic decision-making, there's data extraction. It's the foundational step that can't be bypassed, especially when the sheer volume of data at play is massive.
Consider the time and effort an employee spends on manually transcribing paper-based documents. Now multiply this across all employees and processes. It's not just about the ticking clock; it's about the potential errors that creep in—transcription mistakes, misinterpretations, missed details.
Each error isn’t just a blip; it could translate to significant setbacks, especially in sensitive industries like healthcare, finance, banking, etc.
Take the finance and banking sectors, for instance. Data precision is paramount for these industries. Think about the avalanche of invoices, transaction records, loan applications, and credit reports that flood in daily. Manually processing this deluge not only consumes invaluable time but is also a hotbed for potential errors—misentries, overlooked details, or misinterpretations.
*Enter Intelligent Data Extraction*
It doesn’t just superficially scan documents. It extracts critical data points to attain:
- Enhanced workflow efficiency
- Reduction in errors
- And substantial cost savings
Whether sifting through a dense loan application's fine print or deciphering handwritten transaction notes, this technology captures every little detail. It augments the human touch and ensures that businesses operate more accurately.
It also fares well against traditional, manual methods. Let's draw a comparison:
Manual Data Extraction vs. Intelligent Data Extraction
How Data Extraction Works
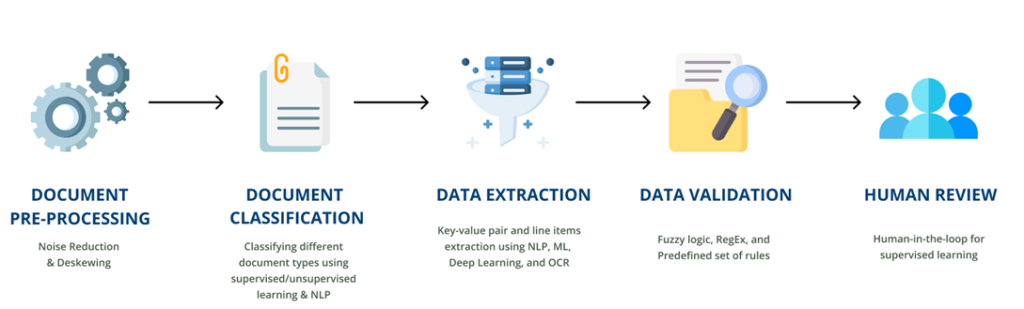
With the right knowledge, the daunting data extraction process can seem more structural and logical. A testament to its significance is the global intelligent document processing market, which stood at USD 1.45 billion in 2022 and is projected to burgeon at a CAGR of 30.1% from 2023 to 2030.
Let's break down this intriguing mechanism step by step.
Step 1. Data Ingestion
Every process starts with raw material. It is the influx of raw data into the system.
Consider a bank onboarding a new customer. The bank gathers digital forms, scanned documents, transaction histories, and more from various channels such as online applications, emails, and mobile banking platforms. These diverse data points, when collectively ingested, set the foundation for the forthcoming steps.
Step 2: Pre-Processing
Data pre-processing ensures only relevant, clean data is used. Unwanted elements are filtered out. This could mean discarding redundant information or converting scanned forms into a more workable digital format, ensuring data consistency.
Step 3: Training the Algorithm
In this phase, machine learning models are 'taught' using sample data, helping them recognize patterns and relationships. Going back to the banking example, algorithms might be trained using thousands of past loan applications, helping the system understand and classify various data fields like "Name," "Annual Income," and "Employment History."
Step 4: Extraction
Here, the actual retrieval of pertinent data points occurs. This involves pinpointing specifics like personal details from a form or amounts from transaction histories. The trained algorithm can now sift through massive datasets, extracting vital details with remarkable accuracy.
Step 5: Validation
No process is flawless. Validation acts as a quality check, confirming the extracted data's correctness. In the bank scenario, a validation tool might cross-check the removed details with pre-defined rules or reference datasets, ensuring, for example, that an account number matches the set format.
Step 6: Continuous Improvement
The world of data is dynamic. Algorithms, once trained, aren't left static. They continuously learn from new data, refining their accuracy. As the bank rolls out new services or changes form structures, the extraction algorithm adapts, ensuring it stays relevant and efficient amidst evolving datasets.
Applications of Intelligent Data Extraction
Before we dive deep into each of the following, here’s a quick look at the benefits of intelligent data extraction across the industries:
By automating and refining the data digestion process, multiple industries can translate scattered data points into actionable insights and optimize their operations. Let's delve into how various sectors are revolutionized by intelligent data extraction:
1. Healthcare
In the intricate landscape of healthcare, precise data extraction is not a luxury but a necessity. Intelligent data extraction streamlines tasks like patient record management, pulling information from handwritten prescriptions to electronic medical records.
Consider a busy hospital—each day, hundreds of diagnostic reports are generated. Automated data extraction can swiftly categorize and store MRI results, blood test details, and physician's notes, ensuring no critical information slips through the cracks.
Beyond administrative tasks, it aids in research by extracting relevant data from vast medical literature, enabling swift treatment plans and better patient care. The end game? Enhanced patient outcomes, reduced administrative burden, and streamlined research processes are all fueled by efficient data management.
2. Observability tools
Observability tools serve as the eyes and ears of digital systems, and application performance monitoring tools become the brain! Here's how intelligent data extraction refines this vision:
- Log Management: Massive log files are generated every second. Data extraction pares these down, highlighting anomalies or patterns and making troubleshooting a breeze. Imagine identifying a recurring system error in seconds, not hours!
- Metrics Optimization: By extracting relevant metrics from vast data, these tools provide a distilled view of system performance, allowing for timely optimizations.
- Real-time Alerts: By identifying and extracting critical incidents from a sea of events, immediate alerts can be triggered, ensuring swift responses to potential system threats.
- User Behavior Analysis: Extracting user-interaction data can shed light on user experience, guiding improvements in interface or system responsiveness.
3. Legal Service Providers
In the meticulous world of law, details can make or break a case. Intelligent Data Extraction brings transformative change to legal services by ensuring no data point goes unnoticed:
- Document Review: Automated extraction speeds up the review of large volumes of documents, pinpointing relevant clauses, dates, or parties involved. Consider the herculean task of sifting through merger agreements—the technology can quickly highlight crucial terms and conditions.
- Contract Analysis: By extracting key terms, renewal dates, or obligations, law firms can provide timely advice to clients about potential risks or renegotiation opportunities.
- Case Research: Extracting precedents from vast legal databases aids lawyers in building robust case strategies and grounding arguments in historical context.
- Client Data Management: Onboarding clients or managing their portfolios? Automated data extraction ensures no pertinent detail, be it an asset or a liability, remains buried in paperwork.
4. Accounting and Taxation
Imagine a bustling tax season. Accountants wade through stacks of receipts, financial statements, and transaction records. Intelligent data extraction comes to the rescue, seamlessly pulling vital figures and ensuring every taxable dollar is accounted for.
Businesses benefit from streamlined invoice processing, where AI swiftly reconciles entries, flags discrepancies, and ensures timely payments. Moreover, for auditors, sifting through financial years of data becomes less daunting as extraction tools highlight anomalies, ensuring full compliance.
5. Banking & Finance
The world of Banking and Finance is filled with pulsating transactions and rapid movements. It demands impeccable precision that's both swift and steadfast.
Envision a scenario: a customer approaches a bank for a mortgage. The bank, swamped with numerous applications, must process these requests rapidly yet thoroughly. With intelligent data extraction, crucial details from application forms—like credit scores, employment records, and asset valuations—are instantly retrieved and assessed.
On the investment side, financial analysts use this tech to sift through market reports. They quickly pinpoint vital details, such as trends in stock performance or key economic signals. Meanwhile, in daily operations, every electronic transfer and credit card use is carefully logged. Any out-of-the-ordinary activity? It's flagged immediately for fraud checks.
Best Practices for Intelligent Data Extraction
For intelligent data extraction (IDE) to reach its full potential, certain best practices should be implemented. These ensure that the extracted data is not only accurate but also actionable. Here's a rundown of practices you can include in this section:
- Quality Over Quantity: Begin with a clear understanding of what data is essential for your specific needs. Prioritize extracting high-quality data over sheer volume.
- Regularly Update Algorithms: Machine Learning models used in IDE can become outdated. Regularly train and update them with new data sets to ensure they remain efficient and accurate.
- Data Verification: Implement a two-step verification process, especially for crucial data, to minimize errors.
- Adaptive Learning: Use algorithms that can learn and adapt from any mistakes made during extraction, refining their accuracy over time.
- Structured Data Storage: Once extracted, data should be stored systematically, making future retrieval and analysis more efficient.
- Ensure Data Privacy: Always stay compliant with data protection regulations, ensuring that personal or sensitive information is encrypted or anonymized.
- Feedback Loop: Establish a mechanism for end-users to provide feedback on the accuracy and relevance of extracted data. This can be used to continuously improve the extraction process.
- Integration with Other Systems: Ensure your IDE system seamlessly integrates with other business systems for smooth data flow and better interoperability.
- Stay Updated: The world of AI and machine learning is rapidly evolving. Stay abreast of the latest advancements and methodologies in data extraction.
- Regular Audits: Periodically review and audit the extracted data for accuracy and consistency. This helps in identifying and rectifying any systemic issues early on.
Are You Reflecting on Data's New Dawn?
They say data reigns supreme. We say, sure, but the true power lies only in meaningful extraction and insightful application. Intelligent data extraction is that which transforms raw data into actionable knowledge.
Equipped with a sophisticated suite of tools, it does more than just identify essential bits of information. It's a continuous learner, refining its methodology with every byte it processes. For contemporary businesses spanning various sectors, the conversation is shifting. It's no longer just about mere data management; it's about ushering in an era of comprehensive organizational automation.
So, as you stand at this informational crossroads, ask yourself: are you merely collecting data, or are you harnessing its full potential?